by WiseOcean | Mar 12, 2024 | AI Digital Transformation, Technology Trends
AI share in the global VC funding keeps increasing, around $20B In February, more than a fifth of all venture funding in February went to AI companies, with $4.7 billion invested in the sector. That was up significantly from the $2.2 billion invested in AI companies in January 2024 and the $2.1 billion invested in February 2023, according to Crunchbase. GPU cloud, humanoid robots, and enterprise AI search got the bigger rounds that stand out.
The deal value in AI startups ends Q4’24 with $22.3 billion across 1,665 deals. Powering these startups are AI-specific datacenters, whose specialized infrastructure and operations are designed to meet the rigors of AI workloads. Some startups in this space are challenging Microsoft, Google, and AWS (The Q4 2023 AI & ML report from Pitchbook). Also, Pitchbook reports that startups in the infrastructure SaaS market are ready to capitalize on the sea change that AI is bringing. The sector saw an uptick in VC funding in Q4 2023—reaching $2.2 billion compared to Q3’s $1.9 billion. Dharmesh Thakker of Battery Ventures predicted the software spend of around $600 billion will increase twofold to threefold over the next five to seven years as AI delivers productivity gains and leads to GDP growth. Let’s borrow the drawing depicted by Elad Gil – the wave of AI product and application building is coming, after the current infrastructure building wave. All are speculations.
The current AI wave is seen as a paradigm shift similar to mobile or the internet. Steve Sloane of Menlo Ventures gave a much longer review of tech waves and lessons (here). Because generative AI is riding on the readiness of software, digital transformation, data, and computing infrastructure already built previously, it has achieved an unprecedented adoption rate. But, that doesn’t equal the revenue generation rate. The most important model of AI is the business model. Menlo Ventures also surveyed more than 450 enterprise executives across the U.S. and Europe and summarized a report – The State of Generative AI in the Enterprise.
Half of the enterprises we polled implemented some form of AI, whether into customer-facing products or for internal automation, prior to 2023. Enterprise investment in generative AI—which we estimate to be $2.5 billion this year—is surprisingly small compared to the enterprise budgets for traditional AI ($70 billion) and cloud software ($400 billion). Enterprises already used AI before. Enterprise execs cite “unproven return on investment” as the most significant barrier to the adoption of generative AI. The median enterprise we surveyed spends more on AI for product and engineering (4.7% of all enterprise tech spend) than on all other departments combined (3.5%). 3 predictions from the report are:
- Despite the hype, enterprise adoption of generative AI will be measured, like early cloud adoption (took years).
- The market will continue to favor incumbents who embed AI into existing products.
- Powerful context-aware, data-rich workflows will be the key to unlocking enterprise generative AI adoption.
We agree with what Anthony Schiavo of Lux Research wrote in The AI revolution will look like a failure. It’s unlikely that the AI use cases generating hype right now will be the ones that drive a lot of revenue. However, LLMs’ ability to make interfacing with digital systems easy for nonexperts might have a meaningful impact on improving the adoption rate. These digital systems could be software, codes, and all physical systems, for example, factory floors, robots, oil field operational systems, defense systems, etc. And, it could enable collaboration between industry experts and those systems. Where will the most value accrue throughout the AI landscape?
Application companies might grow revenues quickly but often struggle with retention, product differentiation, and gross margins from lessons learned previously unless there are moats of adoption such as industry expertise and relationships. All the AI hype and hopes fuel the demands for AI infrastructure – software and hardware. Infrastructure providers are likely the biggest winners in this market so far and in the short term, capturing the majority of dollars flowing through the AI stack.
AI data centers are “picks-and-shovels” for all the above developments, desired explorations with generative AI, and even struggling efforts to find AI business models – all aren’t possible without AI servers. Big funding from VCs or tech titans going into the software infrastructure of generative AI stack in the past year only increased the devastated demand for AI servers and GPUs – hardware is more a bottleneck than software now. Nvidia and Supermicro are enjoying explosive revenue growth and have been breaking records for their stock market caps for quite some time (Supermicro stock goes up over 3X this year, 20X in 2 years, and Nvidia goes up over 2X this year, 4X in 2 years). But both depend on TSMC’s advanced node chip manufacturing and packaging technology. Only 2 months into 2024, TSMC’s revenue has grown around 10% despite the iPhone demand declining, TSMC market cap has grown 50% this year and became the 9th largest enterprise worldwide.
Taiwan has been manufacturing and shipping 90% of global computing servers even before the AI hype. Recently the Taiwan government needs to expand the capacity and area of air cargo operations to support the strong demand for global AI server shipments. TSMC has to keep building the most advanced fabs across Taiwan cities 24/7 (yes, the construction goes 24/7). There are tightly connected ecosystems for semiconductor and hardware manufacturing, that collaborate and respond to demands fast on the small island. Large companies invest in frontier innovations and build technology moats, their small key suppliers can grow fast riding on the wave with high capital efficiency and labor efficiency. These investment opportunities aren’t understood by or accessible to most US or global VCs. If you like to learn more, sign up here.
Thinking of eras — Cisco and HP in the 80’s, Apple and Microsoft in the 90’s, Amazon and Google in the early 2000s, and Facebook and Tesla in the 2010s. In the case of the dot-com bubble, Google and e-commerce allow people faster access to information and goods. Now generative AI is bringing us the next wave, what companies will become the representations of this era, and what opportunities are left for startups?
Anand at CB Insights notes: “The number of deals to AI startups backed by US big tech companies & their strategic venture arms jumped 50% year-over-year. This is the next big super trend, much like the internet and mobile phones, and big tech is committed to not just not missing it but to driving it. They are all in on genAI.” James Mawson of Global Corporate Venturing reminds us: Silicon Valley is the center of the AI world now – but for how long? Abu Dhabi has set up a new technology investment company, MGX, looking to invest up to $100bn in AI infrastructure (data centers and connectivity), semiconductors and core technology and applications such as AI models, software, data, life sciences, and robotics.
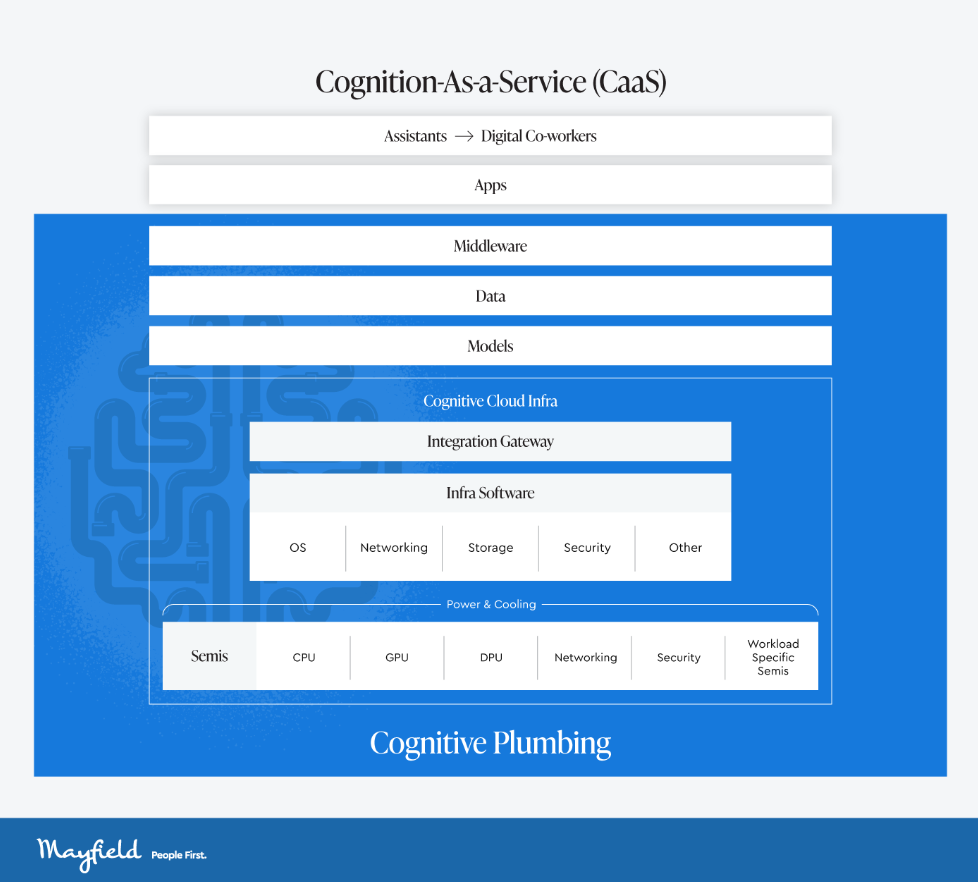
Related:
Abu Dhabi sets up technology investment company MGX amid AI push
Saudi Arabia reportedly in talks with VC firms like Andreessen Horowitz to create mammoth $40 billion AI fund
Gen AI: The next S curve for the semiconductor industry?
by WiseOcean | Jun 19, 2023 | AI Digital Transformation, Major Trends
Key takeaways
- Startup funding is shifting from generative applications to infrastructure solutions to support enterprises build internal tools
- Language use cases dominate enterprise spending and VC investment
- Emerging AI startups with short-term tractions are everywhere, investors need to understand their defensibility and profitability
Generative AI’s fast emerging market
The global generative AI market is expected to reach $42.6 billion in 2023. This sector is the top trend observed from where recent VC funding goes.
The early stage of a tech stack is emerging in generative AI. Hundreds of new startups are rushing into the market to develop foundation models, AI-native apps, and infrastructure & tooling. Models like Stable Diffusion and ChatGPT are setting historical records for user growth, and several applications have reached $100 million of annualized revenue less than a year after launch.
What has now become the critical question: Where in this market will value accrue? Where do VCs bet on? Take a quick look at the sub-sectors with bigger funding: (> $500M in total)
Investment in infrastructure solutions stands out
AI development infrastructure:
- AI applications creation and productionization, e.g. AnyScale
- Run, tune, and deploy generative AI models on any hardware or in the cloud, e.g. OctoML
- Vector database for vendor search, to power semantic search, recommenders, and other applications that rely on relevant information retrieval., e.g. Pinecone
AI data labeling, curation, and model integration:
- Automated data labeling, integrated model training and analysis, and enhanced domain expert collaboration, e.g. Snorkel
- Generative AI architecture to integrate foundation models and all models, full stack for enterprise AI, e.g. Scale AI
In the following chart from Pitchbook, the trend of early-stage VC investment in infrastructure solutions and data platforms going up is very strong against the backdrop of the overall financing climate downturn.
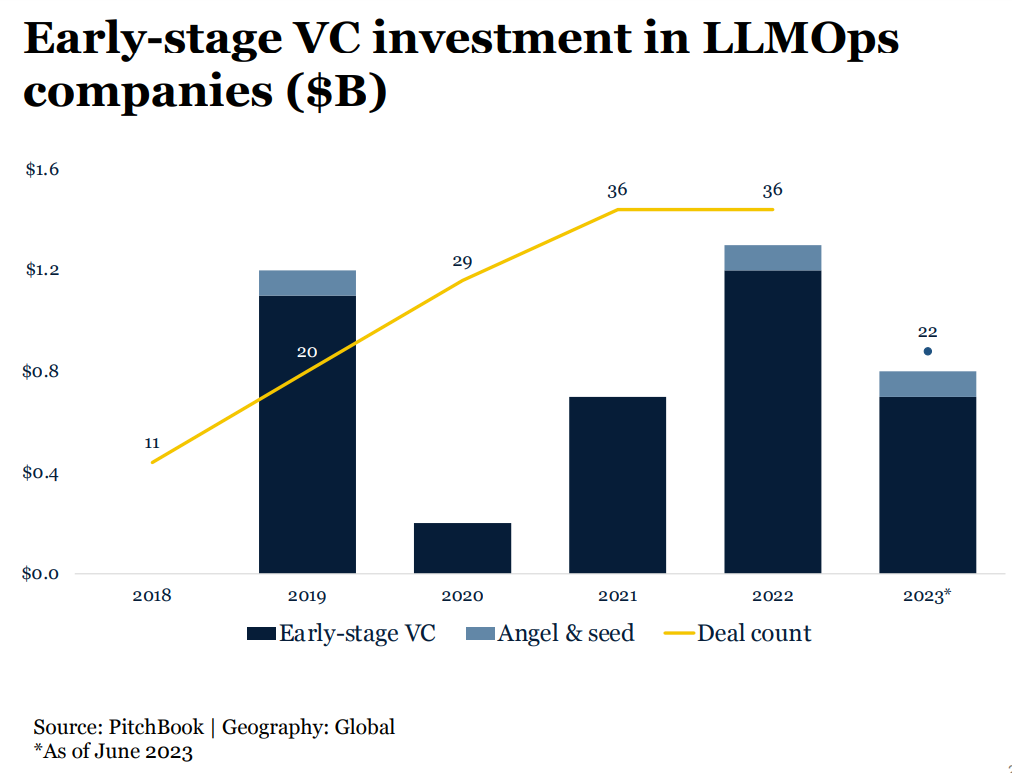
Breaking down the funding flowing into different capabilities in LLM infrastructure shows a lot of new startup categories are forming, including vector databases, prompt engineering, LLM model architecture, LLM orchestration, and LLM deployment, though winners are not yet clear.
Talking about AI infrastructure, everyone knows AI chips underlie all AI computing, but semiconductor is an area with a higher barrier of knowledge and resources for most VCs (read more about semiconductor AI opportunity here), investing in the software stack layer makes sense for them. It’s also a long-term bet, but without starting investing investors don’t get the learning opportunities toward a 10x return.
Language use cases are the new focus in AI applications
Language use cases dominate enterprise spending and VC investment. Applications in sub-sectors like marketing and sales assistance, customer service, infosec, and knowledge management get higher funding.
Visual media and content creation:
- Content creation suite for marketing or the like, e.g. Jasper (B2B or B2C content creation tool without proprietary models)
- Video creation tool, e.g. Synthesia (it makes video production possible for companies without actors, cameras or studios.)
Audio:
- Summarization for meetings, e.g. Fireflies.ai
Coding:
- AI coding assistants, e.g. Kodezi
Natural language interface:
- Chatbot and assistants (interface with customers), e.g. PolyAI, Cresta
- Search and knowledge discovery, e.g. Glean
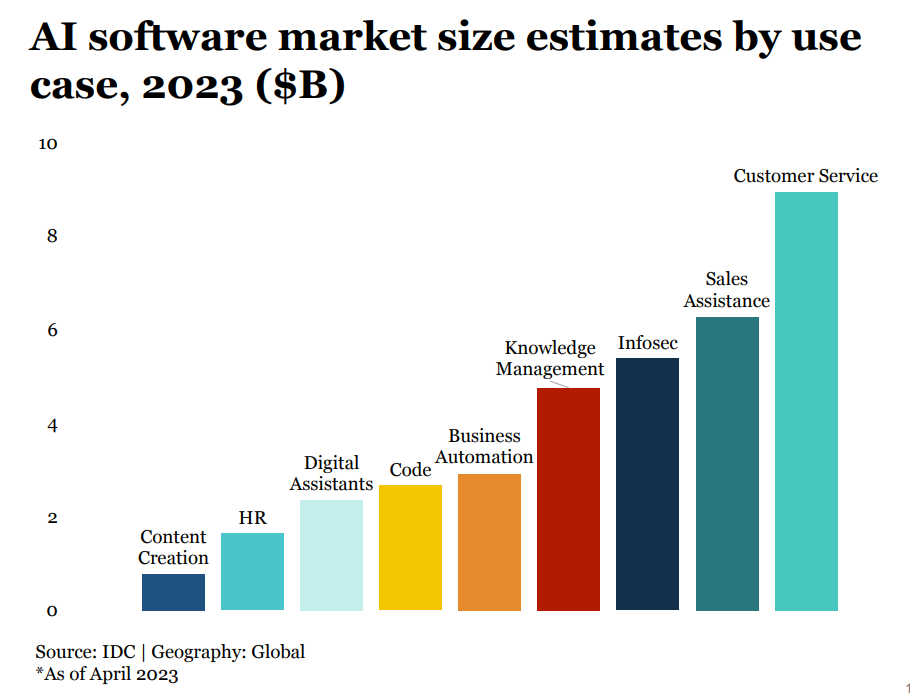
Investors seeking long-term winners
In 2023 January, a16z wrote:
The growth of generative AI applications has been staggering, propelled by sheer novelty and a plethora of use cases. In fact, we’re aware of at least three product categories that have already exceeded $100 million of annualized revenue: image generation, copywriting, and code writing.
However, growth alone is not enough to build durable software companies. Critically, growth must be profitable — in the sense that users and customers generate profits (high gross margins) and stick around for a long time (high retention). In the absence of strong technical differentiation, B2B and B2C apps drive long-term customer value through network effects, holding onto data, or building increasingly complex workflows.
In generative AI, those assumptions don’t necessarily hold true, since the technology itself might change the market dynamics. (read more here)
All moats on deck
Getting OpenAI to focus on a particular corpus and answer questions from that corpus is no longer hard for people with a background in building software solutions. Open-source resources are available to startups as well as incumbents. Emerging AI startups with short-term tractions are everywhere, investors need to understand their defensibility and profitability in either vertical or horizontal positioning. Due diligence and analysis in technology, business, and other kinds of moats are important.
Investors can ask the startup what LLMs they’re using, whether and how they’re fine-tuning, and what their plans are for developing in-house models. Vertical LLMs might replace horizontal LLMs. Insights and data in verticals are a strong moat. Also, investors can ask how well/fast their solutions are adopted by their target audience. User experience and real impact metrics must be delivered, for example, productivity enhancements, and error rate reduction. Even a 1% improvement times a huge business value is worth pursuing.
AI transformation in many verticals is exciting, some verticals might see faster changes than others. But those investors who pursue only fast growth might miss long-term profitability. Behavior change, trust from users, organization change management (workflow change, value chain change…), regulations, physical limitations, relationship building, and other factors might create friction for AI adoption, but overcoming a barrier might become a moat that sticks for long. Last but not least, investors and startups need to watch the market landscape to prevent working on something that tech giants might come in later and take it away.
Reference:
Q2_2023_Emerging_Tech_Future_Report_Generative_AI, Pitchbook
by WiseOcean | Sep 14, 2020 | AI Digital Transformation, Corporate L&D, Talent Digitalization
IBM has been a big blue giant and an AI leader in our memory, its Deep Blue won over the human master at chess in 1997, its Watson won Jeopardy! in 2011, its Project Debater can debate with humans on complex topics. Now IBM’s AI technology has been used in many tough quests from picking stocks to knowledge discovery. How does the Big Blue use AI to transform itself and keep its competitive edge? It all started with transforming its people with personalized development at scale, which isn’t possible without Learning Engineering constructed by experts then automated by AI. Vinod Uniyal, Chief Technology Officer (CTO) of Talent & Transformation at IBM, had a chat with us to share how it works under the hood.
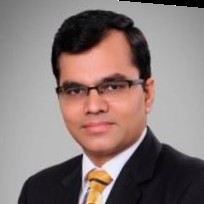
Highlighted quotes:
Five to seven years ago, when we started looking at new technology areas such as cloud, data science, artificial intelligence in a much more meaningful way. That is when we started our transformation, that transformation meant not only changing the way we work, the culture of the organization, the structure of the organization, but also, you know, how do we develop the talent and the skills that we needed for this transformation to be successful. … we also tell our clients that the starting point is understanding the skills that you have.
I already talked about the skills inference, understanding your current skills, then the second step is understanding future skills.
Think about the ways in which you would probably want to ask people — give me what skills do you have, give them a form and ask them “do you have this skill, that skill” — It doesn’t work, it’s a broken process, the only way we can make it work is to automate it by using AI and continuously training. Those algorithms over a period of time will become automated processes, and then it is available all the time. That’s the only way it can work at scale.
There is a big transformation that needs to be made to get to where we are now. The starting point is to build digital literacy, not only in L&D department but also in the entire organization. So everyone has a basic understanding of digital transformation, digital skills, the value of data, how we can leverage data. Having that, the adoption becomes easier. At the same time, the L&D and HR departments look very different today, and they are supposed to look different. The organizations that want to leverage data, AI and all these technologies will need to have certain data skills internally although they can work with service providers.
Jessie: Could you introduce yourself and your role at IBM to us?
My role at IBM is to lead technology for talent development practice, not only for learning and development but also the skills transformation, working at the intersection of AI, Cloud Technologies and Talent. I am very passionate about what I am doing. I am responsible for creating the offering, the Talent Platform and the Ecosystem of IBM learning and skilling solutions for clients and I also work with the clients. I also work with IBM internal HR service team very closely because the solutions we have developed and are being used within IBM, and we take the same solutions to the market. I’ve led the commercialization of many internal solutions. For example, “IBM Your Learning” is a learning SaaS multi-tenant platform where IBM employees go to learn, it was developed for our employees first and then extended to the public and commercial clients later.
We actually see IBM as our first client when we develop learning solutions. I would say that helps us build solutions from a customer-centric mindset, and not to see it as an internal thing. Even our internal employees are our customers. I would like to add that we’ve had many people in our internal IBM HR organization who have actually moved into our consulting practice and the other way around also, so we know exactly what is going on (emerging best practices) in IBM HR.
Jessie: Could you talk about the L&D digital transformation history at IBM?
IBM is almost a 109-year-old company by now. It is still surviving and thriving because IBM has transformed itself over and over again. But if you just talk about the last significant transformation, I think it happened about five to seven years ago, when we started looking at new technology areas such as cloud, data science, artificial intelligence in a much more meaningful way. That is when we started our transformation, that transformation meant not only changing the way we work, the culture of the organization, the structure of the organization, but also, you know, how do we develop the talent and the skills that we needed for this transformation to be successful.
And there have been a lot of strategies that we have used in order to accomplish that kind of skills transformation. I’ll talk about some of those strategies here. So one of the key things there is to understand the skills that we have currently. And this is what we also tell our clients that the starting point is understanding the skills that you have.
Lot of surveys that we have done with the chief executives, most of the chief executives tell us that they really do not know what skills they currently have in their organization. They also struggle in saying that they have all the skills that they need in order to meet their strategic business objectives.
In IBM, since it is such a large scale, you know, we have ~360 thousand employees, what we have done internally is we use skills inference to understand the current skills. What I mean by skills inference is using the digital footprint of the employees to infer the skills from the data, right. So, we use more than 26 data sources as of today, which are internal data sources. Think of your internal intranet, like the collaboration portal where we are posting something, the places where we put our delivery, our internal performance management, those kinds of things where some sort of contributions or deliverables are shared by people, and of course, the training — the kind of training and coaching somebody is undergoing.
So we look at all these data sources and try to infer the skills of the people. So once that has been done, then we present these skills in terms of a skills profile to the employee, we show them that these are the current skills that you have.
Now the second step in skills transformation is to understand the skills that you need for the future. And then the third step is to close that skills gap. And I’ll just repeat it so that we make it a very simple process.
I already talked about the skills inference, understanding your current skills, then the second step is understanding future skills. That is something you can do based on your strategy, interviewing the business leaders, looking at what the trends are, where you want to go and all that. So for IBM, these skills were, probably Cloud, Analytics, AI, Data Science, on the hard skills side, then there are certain ways of working or soft skills, such as Enterprise Design Thinking, Agile, and all other skills like Negotiation, Effective Communication, etc. So basically, we went into certain technology areas and also changed the way we work. You know, how do you work, and for us enterprise design thinking and agile is something which is ingrained into everything we do, including the learning and development department as well. That’s a big change for such a large organization. IBM was supposed to be this big Elephant, the Big Blue, and supposed to move slowly. But in the last five years, I’ve seen tremendous change in IBM.
So, remote work culture, the agile working culture is something that is ingrained. Now, one of the key aspects is once you know that these are the skills that we need, these are the job roles that we need more in the future, you also need to transparently communicate this to your employees, right? So, transparency is very, very important. And I would say once you have that transparency it builds trust, so employees know and understand what the organization actually needs, they will be wanting to learn more, they will say “I want to become a data scientist” or “I want to learn AI or Analytics” or something else…and that has happened in IBM. So, once you have transparently communicated the hot skills and the hot roles, then you should help the employees to understand their skill gaps.
I personally believe in super personalization. The learning that you have available should be personalized to you so that it is relevant for you. Think about Netflix as an example where you get personalized recommendations and you just get hooked on to it. So, similarly learning for us works in the same manner, so, what you are interested in, what people like you are interested in, that is what gets recommended to you. Those AI based personal recommendations actually work like magic in creating that engagement, super engagement in terms of learning.
And I would say it is not only limited to learning, but it goes beyond that because learning doesn’t happen only by consuming digital content, you need to also share it with others, practice what you’ve learned, and then have opportunities to perform tasks and use those skills. So all these elements have to come into play for you to really build those skills.
So that means: learn, share it with others, get coaching from people (like people who are experts in those skills that you are interested in) then get certain opportunities, which we call as internal gig economy so that you can practice those skills in those ad-hoc projects. And once you build those skills, then you get personalized recommendations for jobs which are available internally, so that you can actually move into those different roles.
So this JML (Join-Move-Leave) employee lifecycle, I’ve expanded the cycle to something which I call join, learn, share, grow, and then move internally. That’s the entire life cycle of talent development and skills transformation.
Jessie: Thanks for giving us a whole picture, and simplifying the steps, but the most difficult is the skill modeling. How does IBM do skill inferencing? How to verify the inference? What is IBM Talent Framework? How is it built? How does it work in IBM’s learning ecosystem and support personalized learning? How should an organization use it?
Yeah, I made it very simple. But it is not, the devil is in the details. You’re absolutely right. There are two parts to it. The first step is understanding the skills, you definitely need a base, you need to have a definition of skills, which we call the taxonomy of job skills and roles. And if you have a library, then you get a jumpstart rather than having to build that jobs and skills taxonomy on your own. And we have a library in IBM, which we call IBM Watson Talent Frameworks. So it’s a job and roles taxonomy spans around 16 different industries. 2000 plus skills and thousands of job roles are already defined in that. It is something that we have curated over the last 30 years or so and we continuously keep it updated. So if you start with something like that, you have a jump start. And we can actually help you with that definition of job roles and skills (for your business needs).
And then you can use this definition to infer the skills from the digital footprint data that you have, and match it to the employees. So that will form the base. And once you have it, then you can use these skills to do a lot of other things. And I’ll give you a couple of examples.
One of the things that you can do is tag the learning content that you have with these skills. So if you’re learning content is tagged with the skills then it becomes easier for you to recommend those learning to those people who are interested in jobs linked with those particular skills. And similarly, you can do it with the job roles so you can tag the learning content with the job. So people who are interested in those job roles can get recommendations for that learning content.
Jessie: There’s a crucial link here — you talk about the definition, that’s human languages, how about the machine side? When the machine processes those data, the data from the learning records and also from work behaviors, how do those data represent what skills a person has? There is also an assessment layer — what kind of behavior represents what skills you have.
I can give you some examples that will make it a little bit clearer. So let’s say you have some cases with structured data, most of the cases are unstructured data. One example is you have undergone certain training in AI or Data Science, then you must have some sort of AI or Data Science skills at least foundational skills (according to your learning records). Another example is if you are a salesperson, we look at your sales pipeline and you if have 10 different opportunities pertaining to AI-related solutions, then we can infer that the person who has built that sales pipeline of AI-related solutions must have some sort of AI skills.
The second question is the depth of that skill or the proficiency level of that skill. Most of these things initially would indicate only a foundation level of skill. So if you are making certain contributions to your internal website, you’re sharing something you have created, certain deliverables, that you have shared over a period of time, that will add to your proficiency.
I’ll give you my own example, right. So in the last three months, I have conducted about seven internal sales enablement sessions, which pertain to our Talent Development Offering and Solutions, learning and skills transformation-related solutions that we have. All those sessions were recorded, published, they are available in our learning systems, so people can search for them. So, if I go to my internal website and I look at a solution called Expert Finder. It will identify me as a person who definitely knows something about Talent Development, and AI in Talent, according to his contributions.
So first when you have all the data, you can bring AI algorithms, but then human experts the data scientists will have to get involved, you train the algorithm and make it work in your organization. Once you’ve trained it, then I think you can easily expect 70% ~ 80% kind of accuracy when you run this algorithm at scale. So I’m not saying this is very simple, but it is doable. And when you do it at scale, the benefits are obvious, you don’t want to go back to manual ways (of understanding skills).
Think about the ways in which you would probably want to ask people — give me what skills do you have, give them a form and ask them “do you have this skill, that skill” — It doesn’t work, it’s a broken process, the only way we can make it work is to automate it by using AI and continuously training. Those algorithms over a period of time will become automated processes, and then it is available all the time. That’s the only way it can work at scale.
Brandt: I like your example of taking a presentation or demonstration, which may be harder to track and to account, but digitization makes it easier to track, not only makes it easier to find but, you can track how many people actually find it and use it to give you credit for that expertise as well as for sharing that expertise.
That’s absolutely true. So if you have conducted a session, that record is there, as well as how many people use it, how many people actually like it (like five stars or three stars), all these kinds of ratings are there.
What we have also done is we have created certain Watson based algorithms, which we actually use in content curation, and content optimization. And what I mean by that is… so the content that we have in learning is in different formats, right? It could be SCORM based content, it could be Word, PDF, PowerPoint, etc.. The algorithms actually help us TAG this content automatically. As learning professionals, the instructional designers are usually Tasked with this (tagging of the content) — okay, you have created this content, put certain tags into it so that somebody can find this content. People would generally put 2-3 mandatory tags and be done with it. Does it make it discoverable? It does not. So the algorithm actually reads the content, almost as if a human being is reading it. And it brings out the key phrases from the content and other tags. And once we put this into the systems, it makes the content discoverable. It makes it personalized because now those key phrases and tags are available for any type of content that you have. So that is one example.
The second thing that we do with the same solution is to break the content into smaller parts. We call it chunking — chunking based on the logical composition of the content, we can break the content in terms of the syntactic structure of the content, or we can look at the semantic attributes as well. So syntactic is easier because you have a structure (e.g. Table of contents) already in place. But if there is no structure there, no table of content, it can read the content. And as soon as the topic changes, it breaks it there into a different segment (chunk). We have seen accuracy levels of almost 80 to 90% in these cases, which is fascinating. And then we expanded the same algorithm to do a lot of other things that I was talking about, — tagging the learning content with those skills, tagging the learning content with jobs, and job roles.
Based on the jobs and skill taxonomy you run all other things. In most enterprises, it is a mess, right? They don’t have good data there. You may have a lot of jobs that are duplicate, which actually are similar jobs but have different names, jobs requiring similar skills. Unless you can clean that data, curate that data, you will not be able to use it. So, what we do with these AI algorithms is look at the jobs and the definitions, and the algorithms actually tell us what is the match between these two different job roles or 10 different job roles. That actually helps the human beings who are working on it. Now they can see if this job role and that role is like 90% similar, and ask “can we get rid of one of them?” So, that’s the way we are using AI to augment human consultants.
I can talk a bit about our strategic workforce planning approach. There are seven B’s.
- The first is the Business strategy which links to talent demands.
- For the Talent Acquisition, you can either Buy permanent resources, or you can Borrow contingent resources (temporary resources).
- For the Talent Evolution, you can Bind the resources — that means move the resources from one part to another and retain them.
- For the Talent Management, you can Bounce — meaning exit the resources that you don’t need, and Build — develop the resources.
- For the Talent Augmentation, you can use Bots – this is very important, we think of Bots as resources whether it is automation or AI.
- Seventh B is Balance, continuously balancing your workforce rather than keeping it static and at most an annual planning exercise.
This is our thinking about strategic workforce planning, this is how you can build an agile workforce that will continuously help you align with the changing external environment.
We have the project and learning marketplace, it’s sort of a talent marketplace within the organization where we have talent at one end (their skills), and then the opportunities at the other end — whether they are temporary opportunities or permanent opportunities. So there’s a continuous matching of talent with those opportunities and coaching opportunities, project opportunities, permanent job opportunities, all that happening on a continuous basis. That’s what I call a talent marketplace. It also doubles up as the place for you to crowdsource, you know, talent and innovation. If you have challenges that you want to throw at your people, it is the way in which you can actually build teams on an ongoing basis. You don’t have to have permanent roles to fill those projects, you can create projects and find the talent, which probably is residing in some other departments and build those agile project teams to get things done and then move on.
Cognitive chatbots are built into the entire ecosystem. So, there you can talk to a chatbot and ask about the learning recommendations, you can ask any questions that are linked to learning or career or skills and all that.
We use AI algorithms for content curation, content personalization, I’ve talked about them. Content harvesting is again a very interesting application. If you have a lot of Informal sources within the organization, you can actually use the algorithms to source the content or harvest the content. You give these terms, objectives to the algorithms that I want to find content on, let’s say data science, it’s gonna find the content on data science from all the different sources that you will assign to it and bring it in front of you. So instead of human beings doing that kind of harvesting, we can use algorithms to do that content harvesting.
About the credentials, there is a combination of open digital badges. In IBM, digital badges have been such a tremendous success in the last couple of years. The numbers are mind-boggling, actually. In 2019, IBM has consumed 27 million hours of learning and issued three million badges, and in recent weeks, we have been issuing almost 80,000 badges every week. The numbers have gone up drastically during the pandemic. We have our technology behind the digital badges to issue them automatically.
We are currently working with several institutions to set up Blockchain-based credentials.
Jessie: Many enterprises don’t have good data so they hesitate to pursue personalized learning, what will you suggest to them to get started? What skill do the L&D department, business managers, and employees need to use IBM’s “cognitive learning” solution and those analytics insights?
There is a big transformation that needs to be made to get to where we are now. The starting point is to build digital literacy, not only in L&D department but also in the entire organization. So everyone has a basic understanding of digital transformation, digital skills, the value of data, how we can leverage data. Having that, the adoption becomes easier. At the same time, the L&D and HR departments look very different today, and they are supposed to look different. The organizations that want to leverage data, AI and all these technologies will need to have certain data skills internally although they can work with service providers.
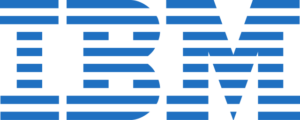
Related References
Rebooting work for a digital gap
In addition to the new approach to performance management, talent management at IBM now includes a personalized learning platform and a personalized digital career adviser.
The platforms use data to infer which skills employees have and connect them with learning to build those skills that are increasingly in demand.
More predictive and prescriptive insights will be transmitted directly to managers and employees at the moment they’re needed most, embedded in the workflow.
(Jessie: the way IBM has engaged its employees to participate in the transformation journey with their voices isn’t possible without the help of AI, for example, Watson text analytics)
The enterprise guide to closing skills gap
IBM Talent Framework
IBM Skills (free resources for learning tech skills and for educators)
IBM awards its three millionth digital badge (and disrupts the labor market)